Eric Zhao
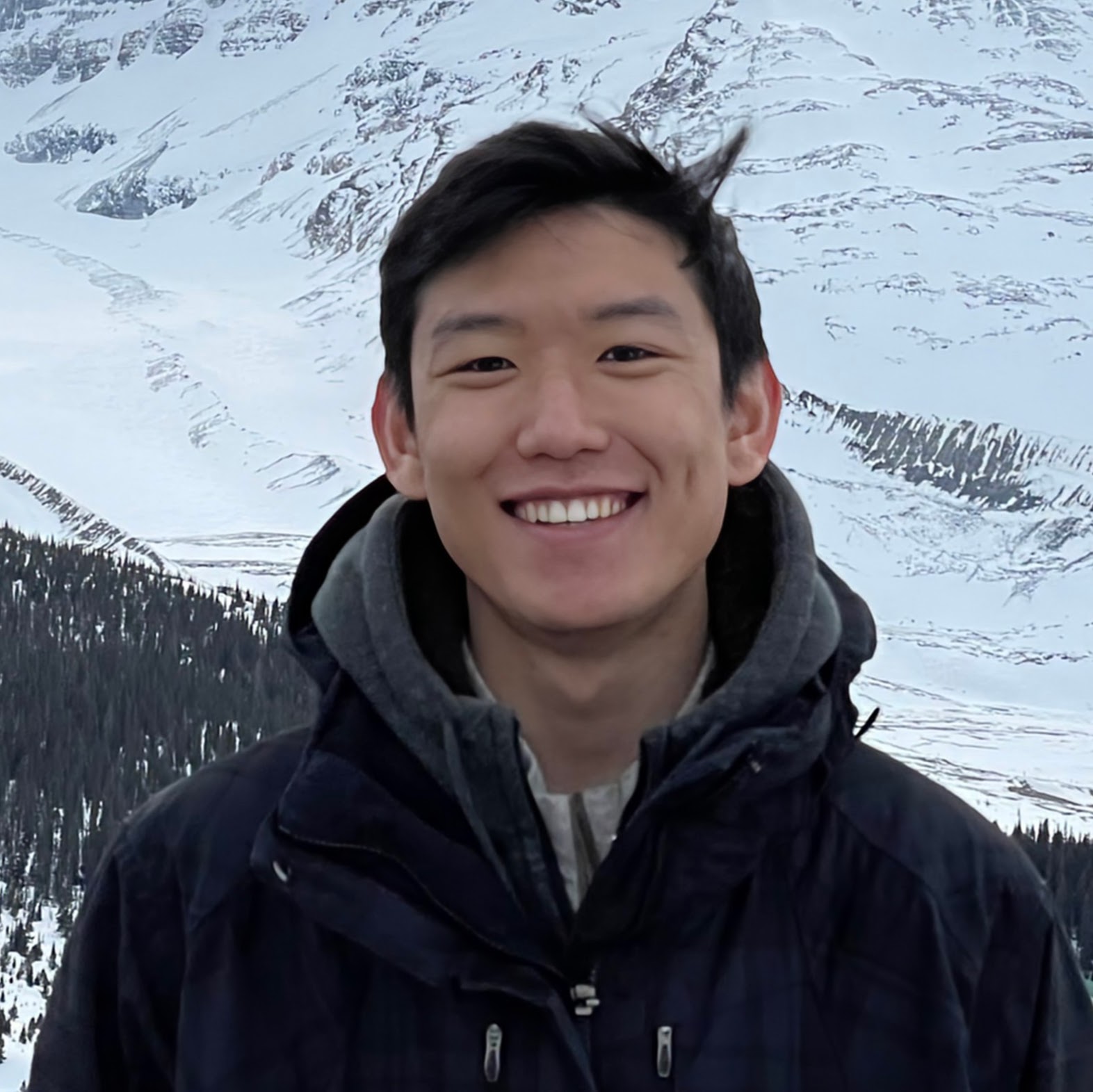
BWW 8070-14
2121 Berkeley Way
Berkeley, CA 94704
I’m a third-year computer science PhD student at UC Berkeley and concurrently a visitor at Google Research and Simons Institute. I’m advised by Nika Haghtalab and Michael I. Jordan, and a member of the Berkeley Artificial Intelligence Research Lab and the CS Theory group.
My research develops algorithms and mathematical foundations for multi-objective machine learning. I am particularly interested in multi-objective learning as a means of (1) understanding the emergent capabilities of large language models and (2) designing automated decision-making systems in marketplaces and other multi-agent environments.
I’ve previously worked on large language models at Google Research, automated mechanism design at Salesforce Research, algorithmic fairness at Nvidia Research, and self-driving cars at Uber. I received my bachelor’s degree from Caltech (California Institute of Technology) in 2020.
My CV can be found here.